
FAST is an open-source framework developed by researchers at the Norwegian University of Science and Technology (NTNU) and SINTEF. The main goal of FAST is to make it easier to do high-performance processing, neural network inference, and visualization of medical images utilizing multi-core CPUs and GPUs. To achieve this, FAST use modern C++, OpenCL and OpenGL, and neural network inference libraries such as TensorRT, OpenVINO, TensorFlow and ONNX Runtime.
Get started
See installation instructions for Windows, Ubuntu Linux and macOS.
To start using the framework, check out the C++ tutorials or the Python tutorials.
Learn best by example? Check out all the examples for C++ and Python.
Need help? Post your questions on the Discussions page or use the Gitter Chat.
Main features
- Data streaming – Processing pipelines in FAST can handle both static and dynamic/temporal data without any change to the code. FAST can stream data from movie files, your webcamera, an Intel RealSense camera, a sequence of images and even directly from ultrasound scanners such as Clarius.
- Deep learning – FAST provides a common interface for neural networks supporting different model formats (ONNX, protobuf, SavedModel, OpenVINO, UFF) and backends (Google TensorFlow, NVIDIA TensorRT, Intel OpenVINO, Microsoft ONNX Runtime), making it possible to create real-time neural network pipelines.
- High-level data management – Data objects in FAST represent data, such as an image, on all processors. FAST keeps data coherent across the different storage areas thereby removing the burden of explicit memory handling from the developer.
- Wide data format support - FAST supports several data formats (DICOM, metaimage (MHD), regular jpg/png/bmp images, videos, HDF5, VTK polydata, whole slide images, ultrasound file format) and data types (images 2D and 3D, grayscale and color, image pyramids, surface mesh, vertices, lines, text ++).
- High performance algorithms – FAST has several high performance parallel OpenCL implementations of common algorithms, such as marching cubes surface extraction, Gaussian smoothing, non-local means, block matching tracking and seeded region growing.
- Fast concurrent visualization – Rendering and computation are done in separate threads to ensure smooth responsive visualizations. Several types of visualizations are supported both 3D (mesh, point, line, image slice and volume rendering) and 2D (2D image, image slice and segmentation/label rendering, whole slide image (WSI) pyramids).
- Interoperability – FAST can be used with python and can also be easily integrated into existing Qt applications.
License
The source code of FAST is licensed under the BSD 2-clause license, however the FAST binaries use and are linked with many great third-party libraries which has a number of different open source licenses, see the licenses folder in the release for more details.
Research
FAST has been described in the following research articles. If you use this framework for research please cite them:
FAST: framework for heterogeneous medical image computing and visualization
Erik Smistad, Mohammadmehdi Bozorgi, Frank Lindseth
International Journal of Computer Assisted Radiology and Surgery 2015
High Performance Neural Network Inference, Streaming, and Visualization of Medical Images Using FAST
Erik Smistad, Andreas Østvik, André Pedersen
IEEE Access 2019
Build
To setup and build the framework, see the instructions for your operating system:
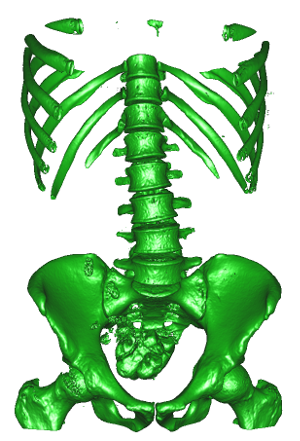
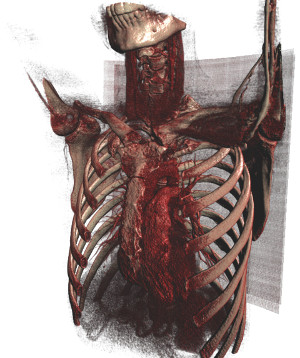
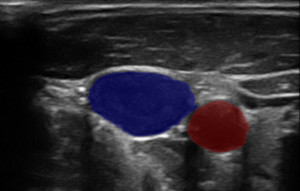
